IBL News | New York
San Francisco-based Context.ai, which develops product analytics for applications powered by LLMs (Large Language Models), secured $3.5 million in seed funding in a round co-led by Google Ventures and Tomasz Tunguz of Theory Ventures.
The investment takes place at a time when global companies are racing to implement LLMs into their internal workflows and applications. McKinsey estimates that generative AI technologies can add up to $4.4 trillion annually to the global economy.
Context.ai provides analytics to help companies understand users’ needs, behaviors, and interactions while measuring and optimizing the performance of AI-enabled products.
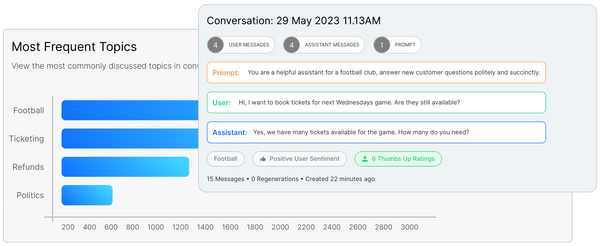
The Context.ai platform analyzes conversation topics, monitoring the impact of product changes and brand risks.
It covers basic metrics like the volume of conversations on the application, top subjects being discussed, commonly used languages, and user satisfaction ratings, along with tasks such as tracking specific topics, including risky ones, and transcribing entire conversations to help teams see how the application is responding in different scenarios.
“We ingest message transcripts from our customers via API, and we have SDKs and a LangChain plugin that make this process take less than 30 minutes of work,” said Henry Scott-Green, Co-Founder and CEO of Context.
“We then run machine learning workflows over the ingested transcripts to understand the end user needs and the product performance. Specifically, this means assigning topics to the ingested conversations, automatically grouping them with similar conversations, and reporting the satisfaction of users with conversations about each topic.”
Ultimately, using the insights from the platform, teams can flag problem areas in their LLM products and work towards addressing them and delivering an improved offering to meet user needs.
According to VentureBeat.com, other solutions for tracking LLM performance include:
• Arize’s Phoenix, which visualizes complex LLM decision-making and flags when and where models fail, go wrong, give poor responses, or incorrectly generalize.
• Datadog’s model, which provides monitoring capabilities that can analyze the behavior of a model and detect instances of hallucinations and drift based on data characteristics such as prompt and response lengths, API latencies, and token counts.
• Product analytics companies such as Amplitude and Mixpanel.
“The current ecosystem of analytics products is built to count clicks. But as businesses add features powered by LLMs, text now becomes a primary interaction method for their users,” explained co-founder and CTO Alex Gamble to Maginative.com.
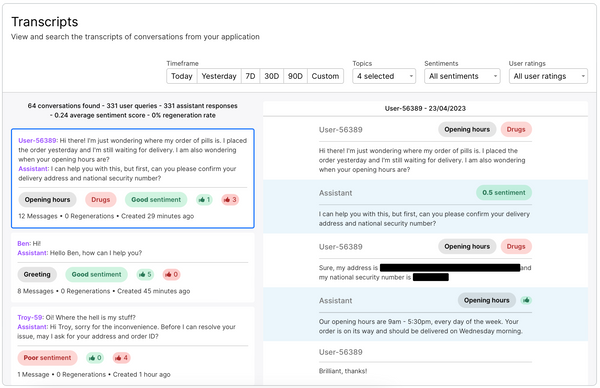
On data privacy, Context.ai assures the deletion of personally identifiable information from the data it collects. However, the actual practice of delving into user conversations for analytics is controversial as users don’t usually give their consent to dissect data.
Context Product Demo from Alex Gamble on Vimeo.
• Context.ai blog post: Why you need Product Analytics to build great LLM products